Matrices and vectors math for AI with Python examples
Vectors and Matrices math is one of the basic tools used for AI. There’s a lot you can do with vectors (and matrices, tensors, and so on). Linear algebra is a good place to master vectors, but let’s take a look at popular basic stuff that can help in a lot of cases.
What are vectors and why do we use them
Vector is a point in (some) space, which we are “looking at” from (some) point (usually zero point). In simple 2-dimensional space, vectors look like this:
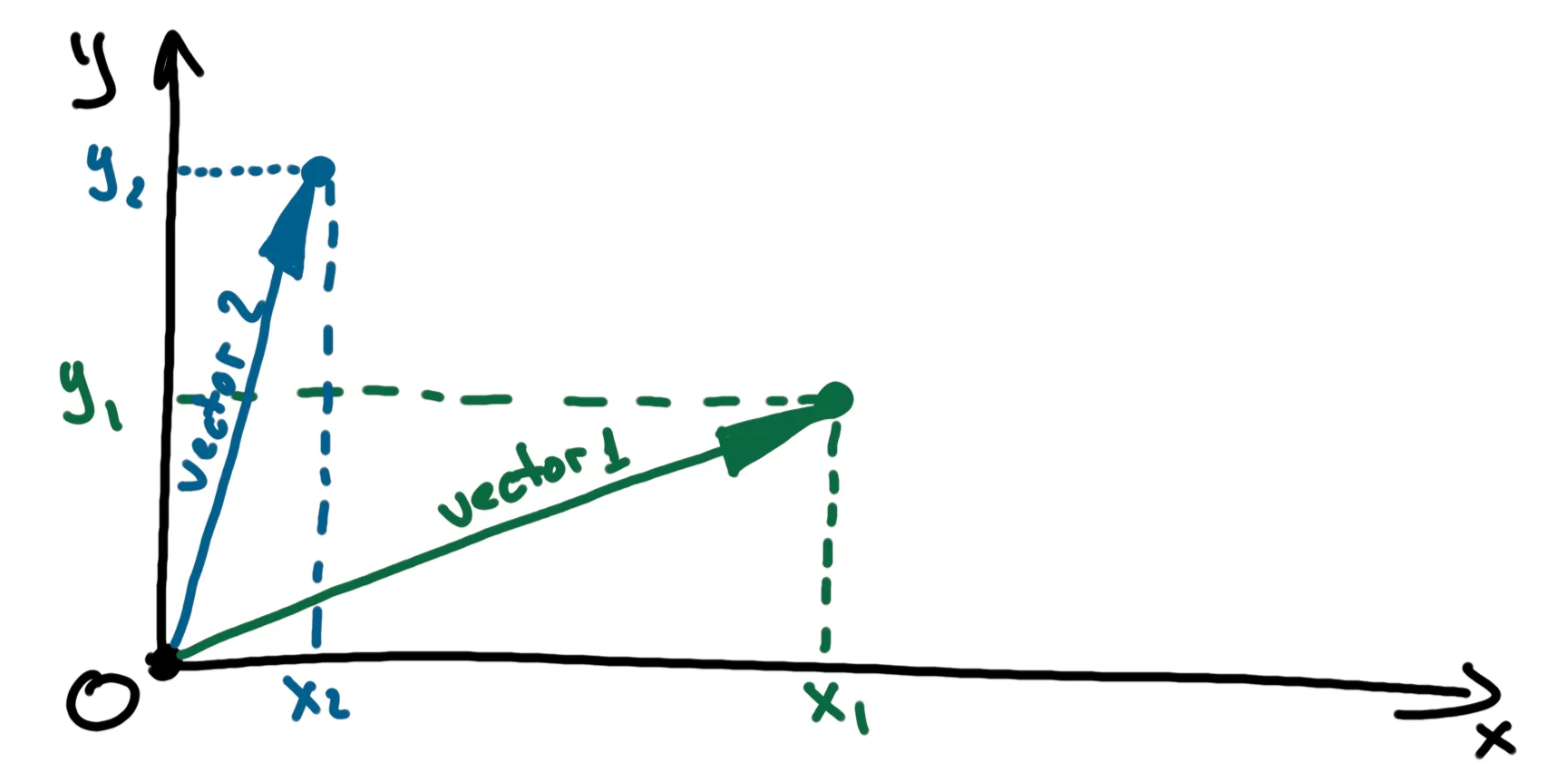
We have 2 vectors here. Each is described by 2 coordinates — x
and y
. Vectors are usually written down as:
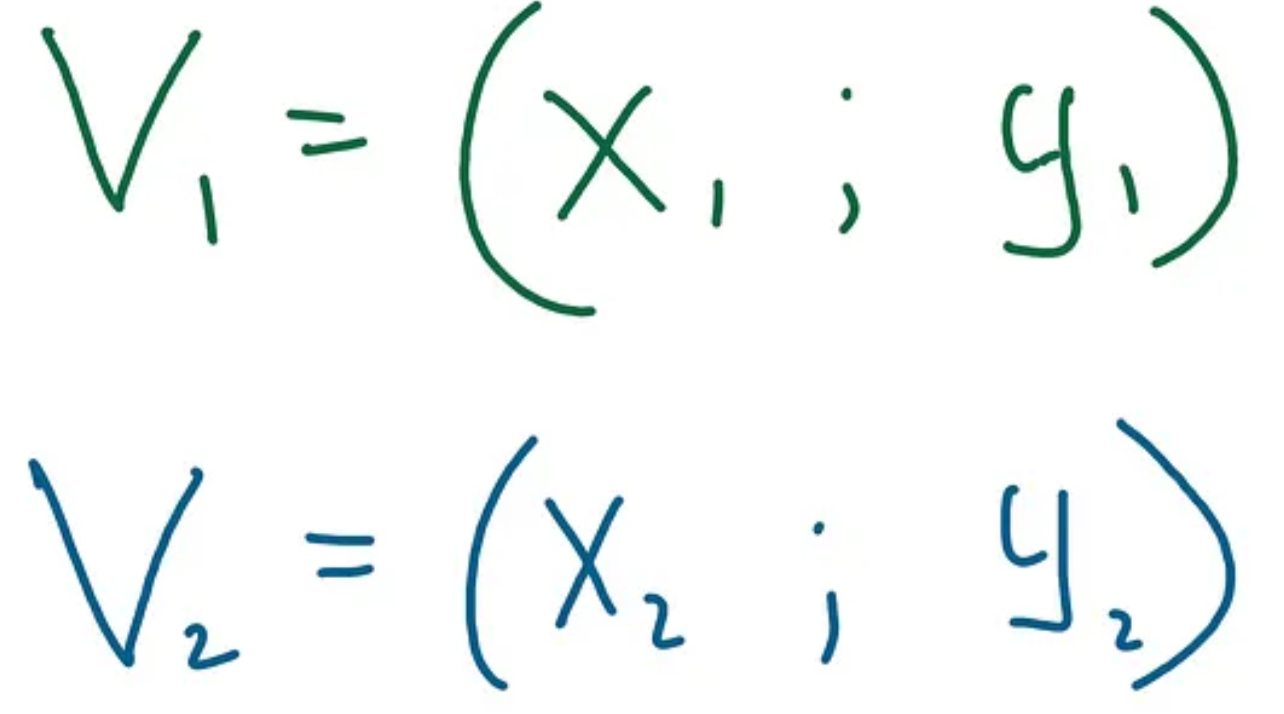
Vectors can have more than 2 dimensions. For example, 3-dimensional vector will look like this:
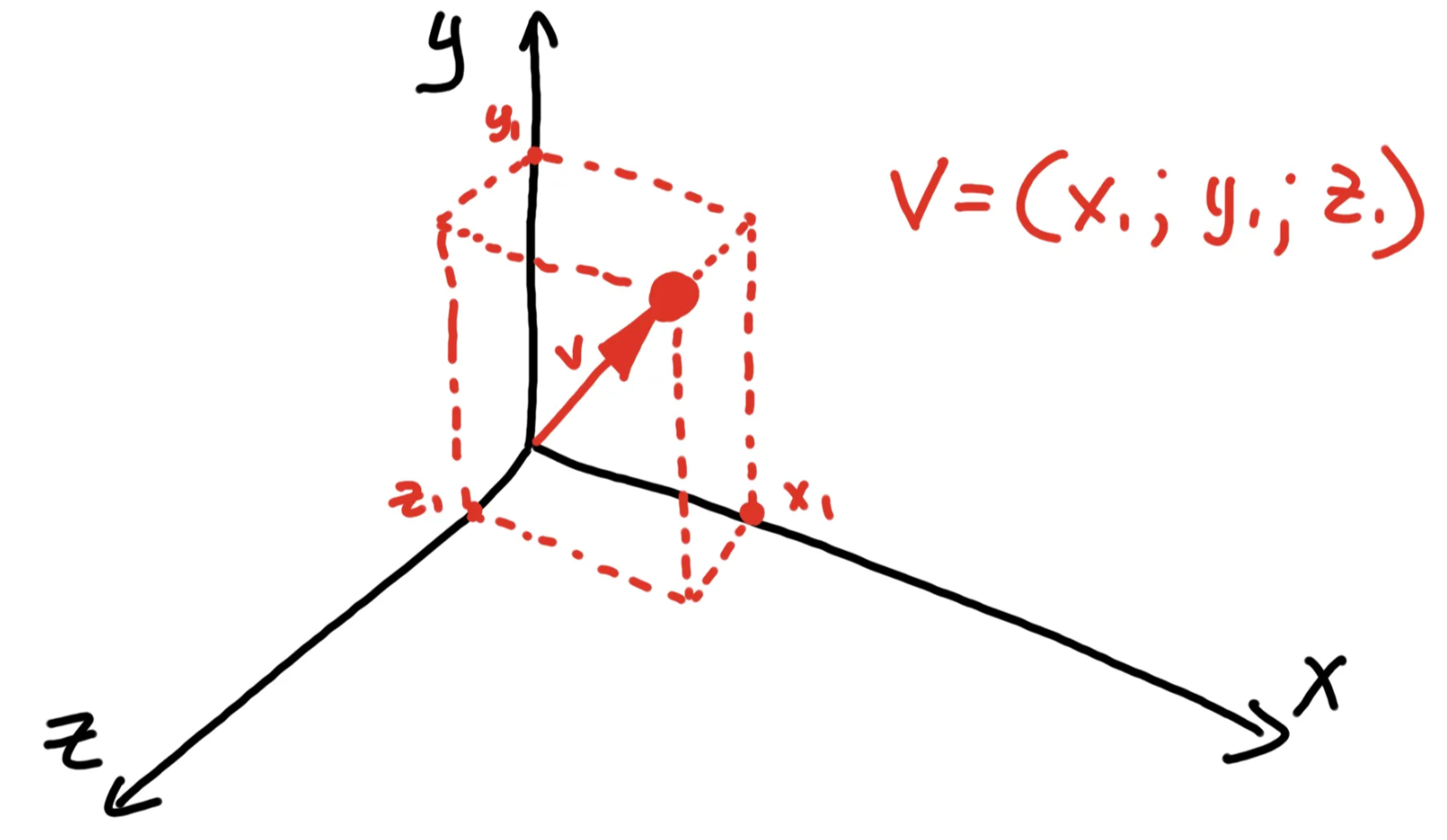
More dimensions will be harder to draw, but we basically can have any number of coordinates for vector:
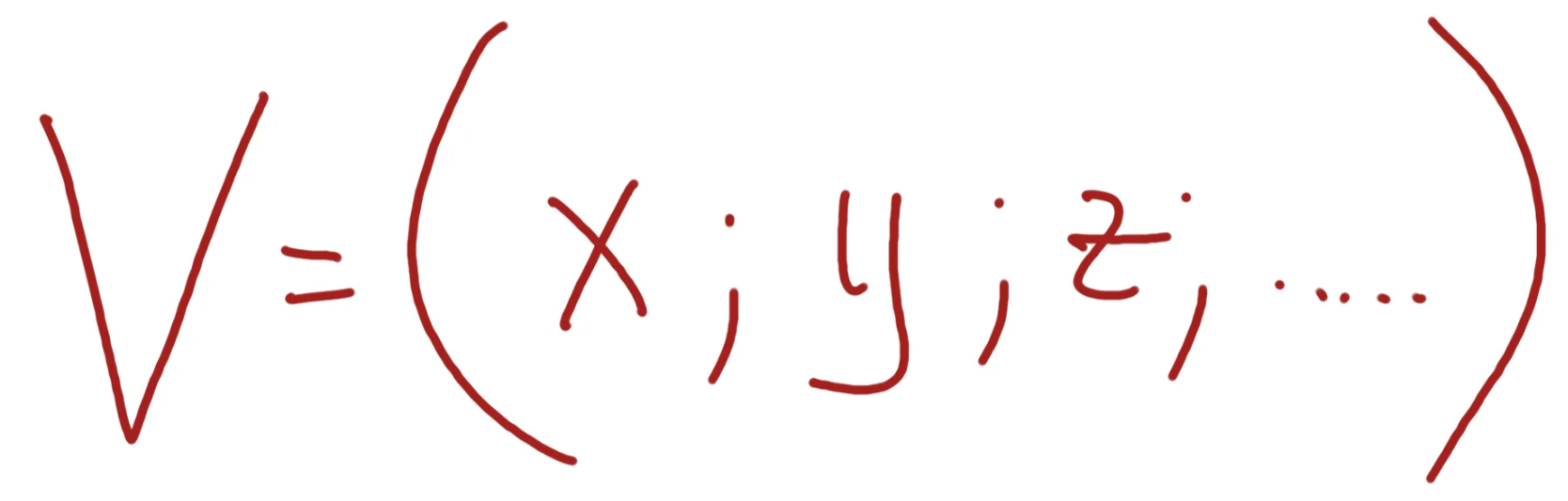
Now let’s imagine, that we have a set of people that we want to analyze. Each person is described by several features - age, weight, and height. We can, in the language of math, say that we have a set of vectors in 3-dimensional space:
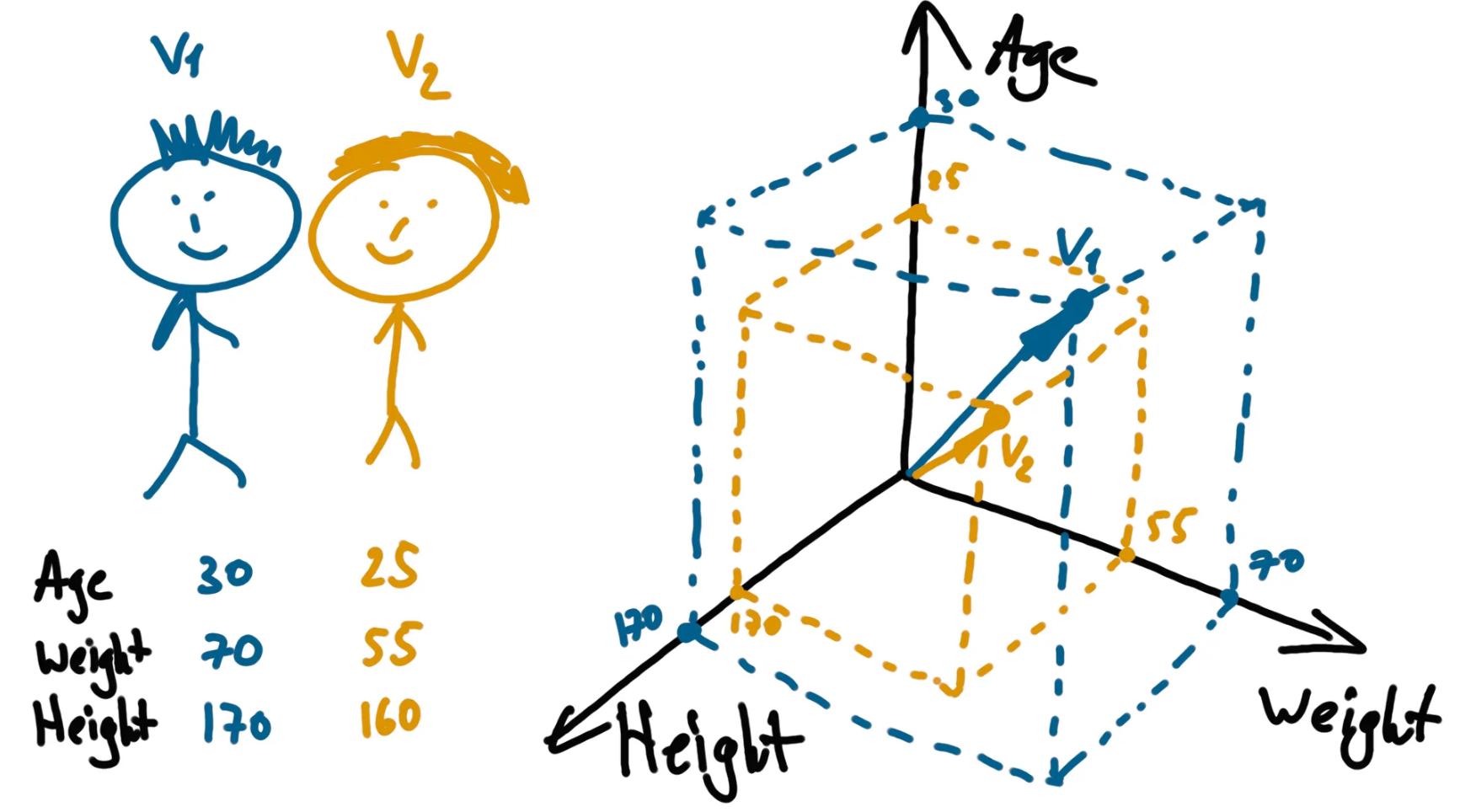
So it’s easy to write down a list of observations (people in our case) with features (age, width, height in our case) as vectors:
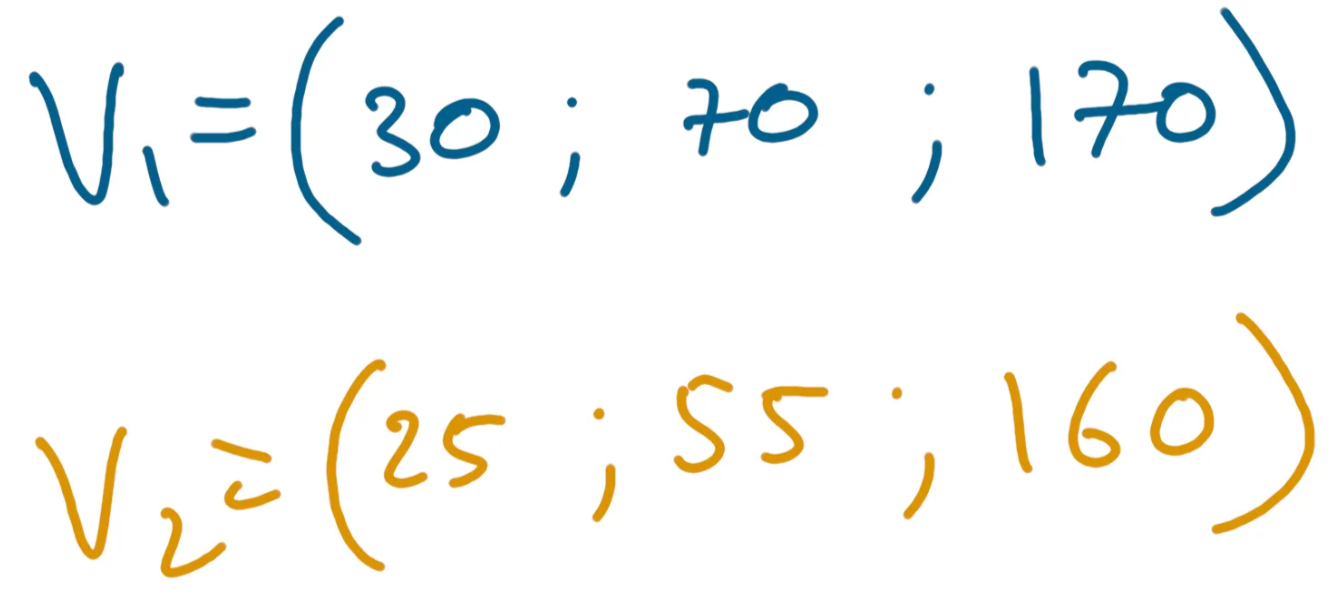
And this means we can apply vector math to analyze our data. And vector math has a lot of powerful tools. But before looking at math operations, let’s discuss another structure, called a matrix.
Matrices are also vectors
Matrix — is a set of vectors. Easy. Let’s imagine a vector, called M, which has V1
and V2
(two vectors from the previous example) as coordinates:
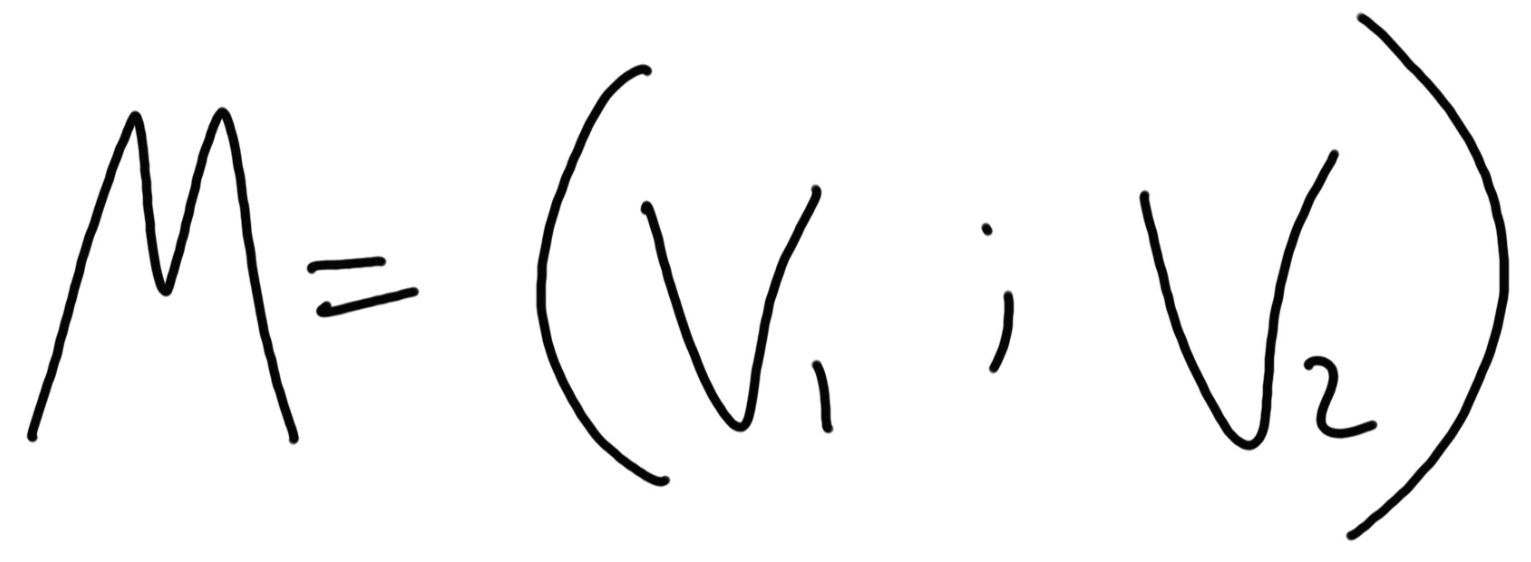
This is called matrix. We can use another, more popular, form to write the matrix down:
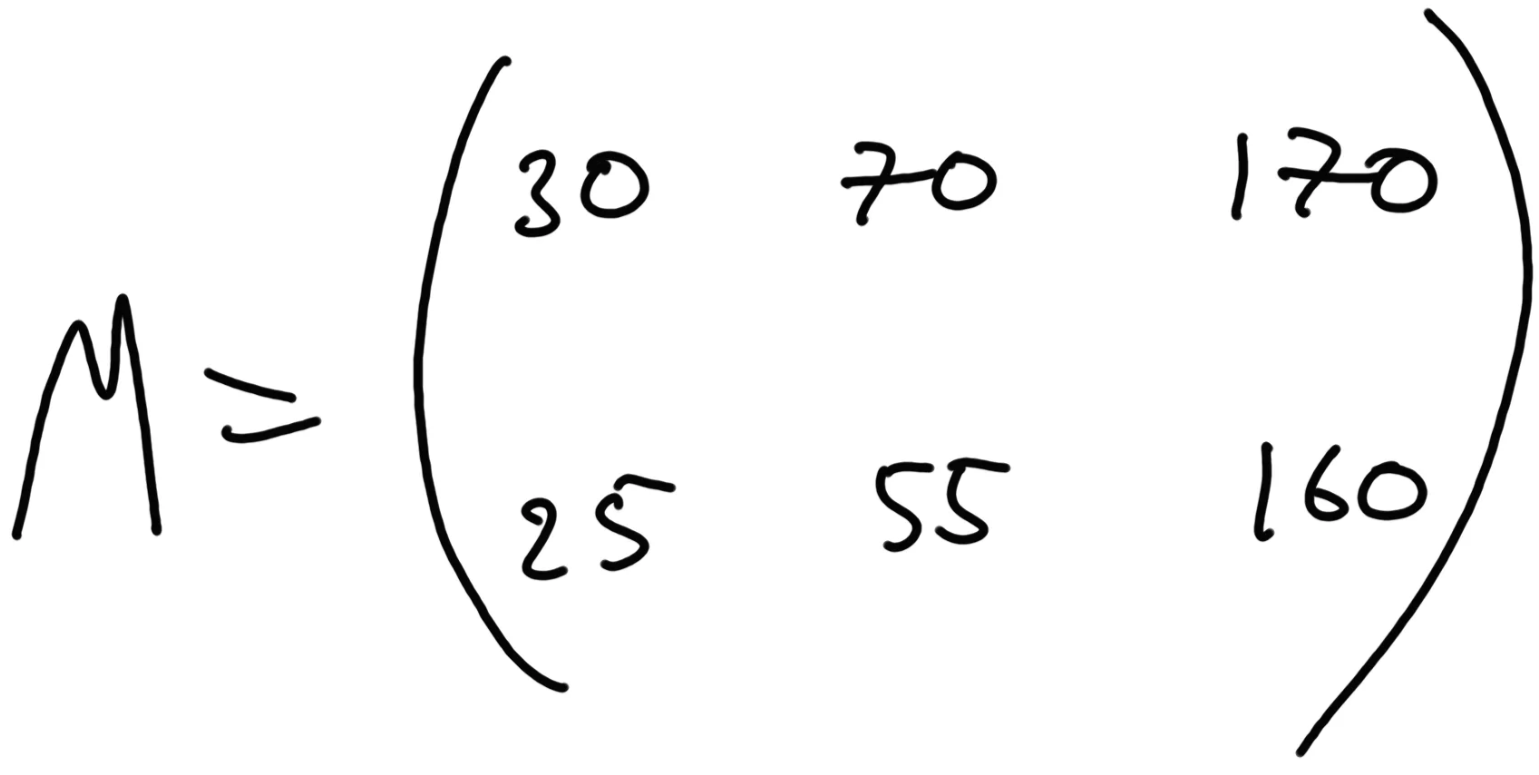
We say that this matrix has 2 dimensions — 3 columns and 2 rows, so the form of the matrix is 3x2
. Matrices can have any number of dimensions and be of any form. Example of 3-dimensional matrix 2x2x3
:
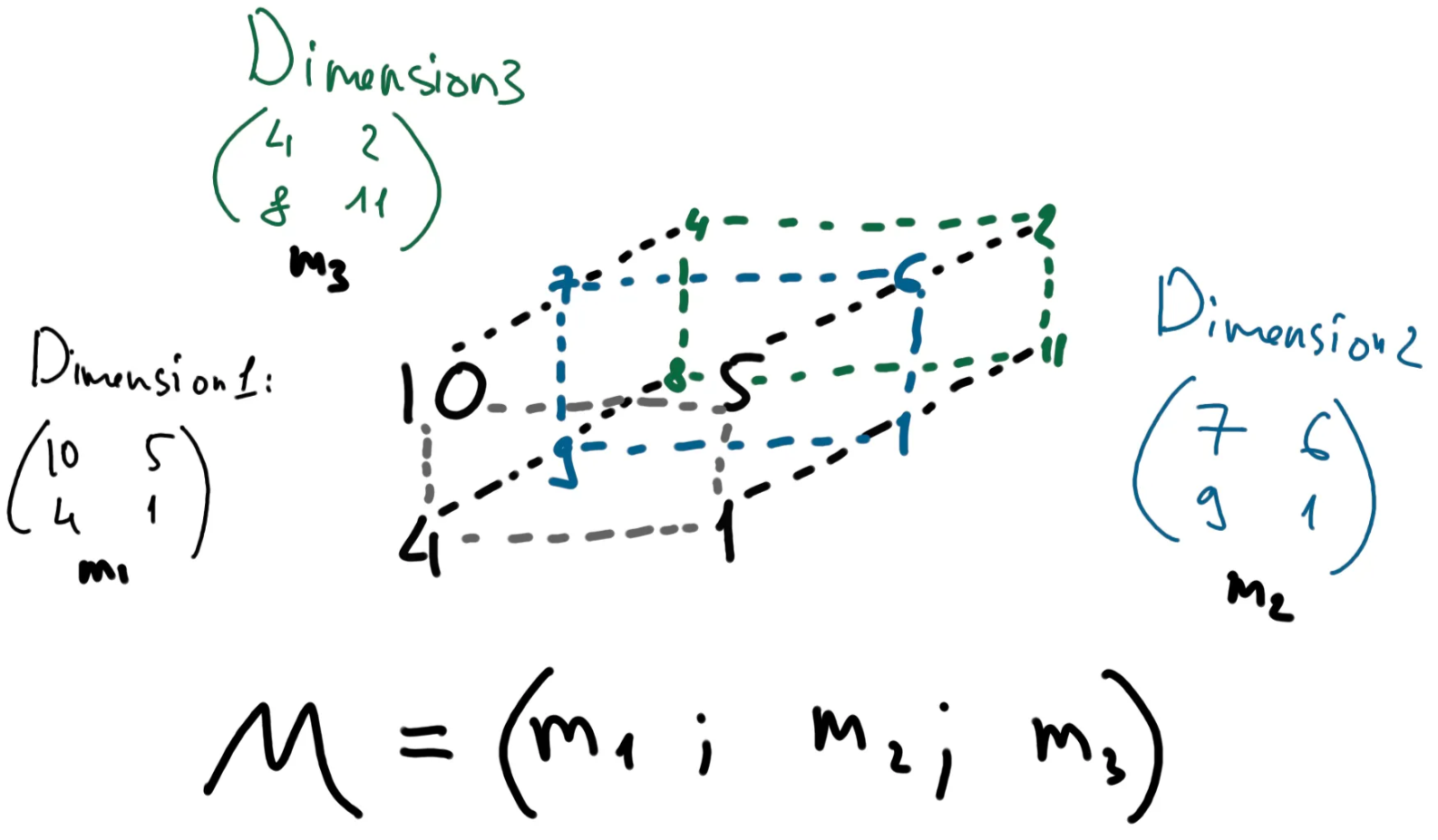
So, a 3-dimensional matrix is a vector of 2-dimensional matrices. And, as we remember 2-dimensional matrix — is a vector of vectors. So 3-dimensional matrix is a vector of vectors of vectors. And this means, everything comes down to vector math.
Let’s look at some basic vector/matrices operations.
Euclidean norm (distance)
Euclidean norm (or distance) is a way to calculate the mathematical “length” of a vector. It is calculated as:
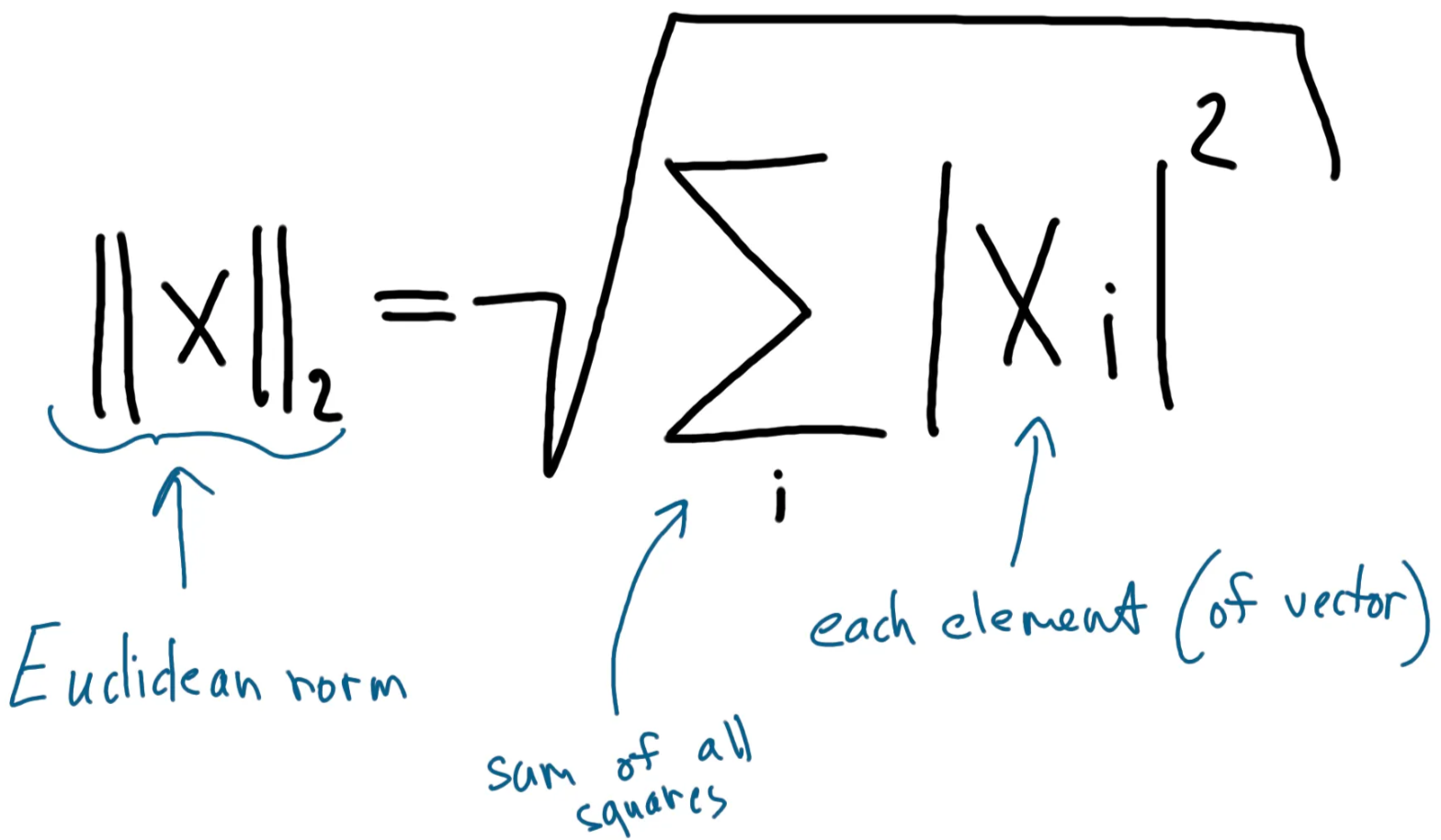
In Python, this can be calculated using the Numpy package. Let’s find euclidean distance for a sample vector (1, 2, 3)
:
import numpy as np
a = np.array([1,2,3])
dist = np.linalg.norm(a)
print(dist)
3.7416573867739413
import numpy as np
— import Numpy modulenp.array([1,2,3])
— define Numpy arraynp.linalg.norm
— return Euclidean norm for a given array
Adding vectors or matrices
To add 2 vectors, we have to add all corresponding elements of our vectors:
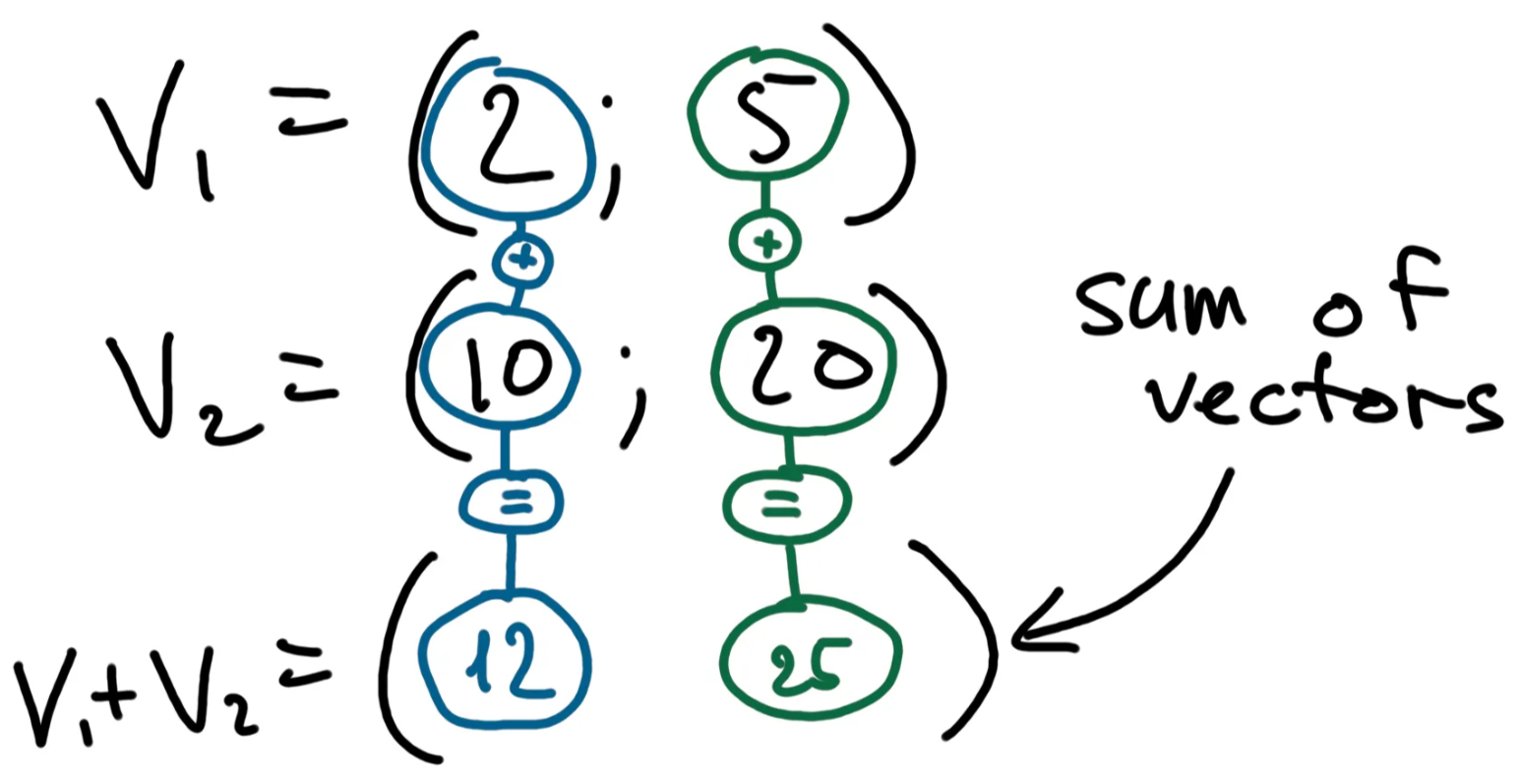
In Python, you can just use the standard +
operator to add Numpy-defined vectors:
import numpy as np
a = np.array([1,2,3])
b = np.array([10,20,30])
sum = a + b
print(sum)
[11 22 33]
a + b
— we can use+
with Numpy arrays to add themsum
— will also be a Numpy array
Because, as we know, matrices are also vectors, to add 2 matrices we have to add each element of our matrices:
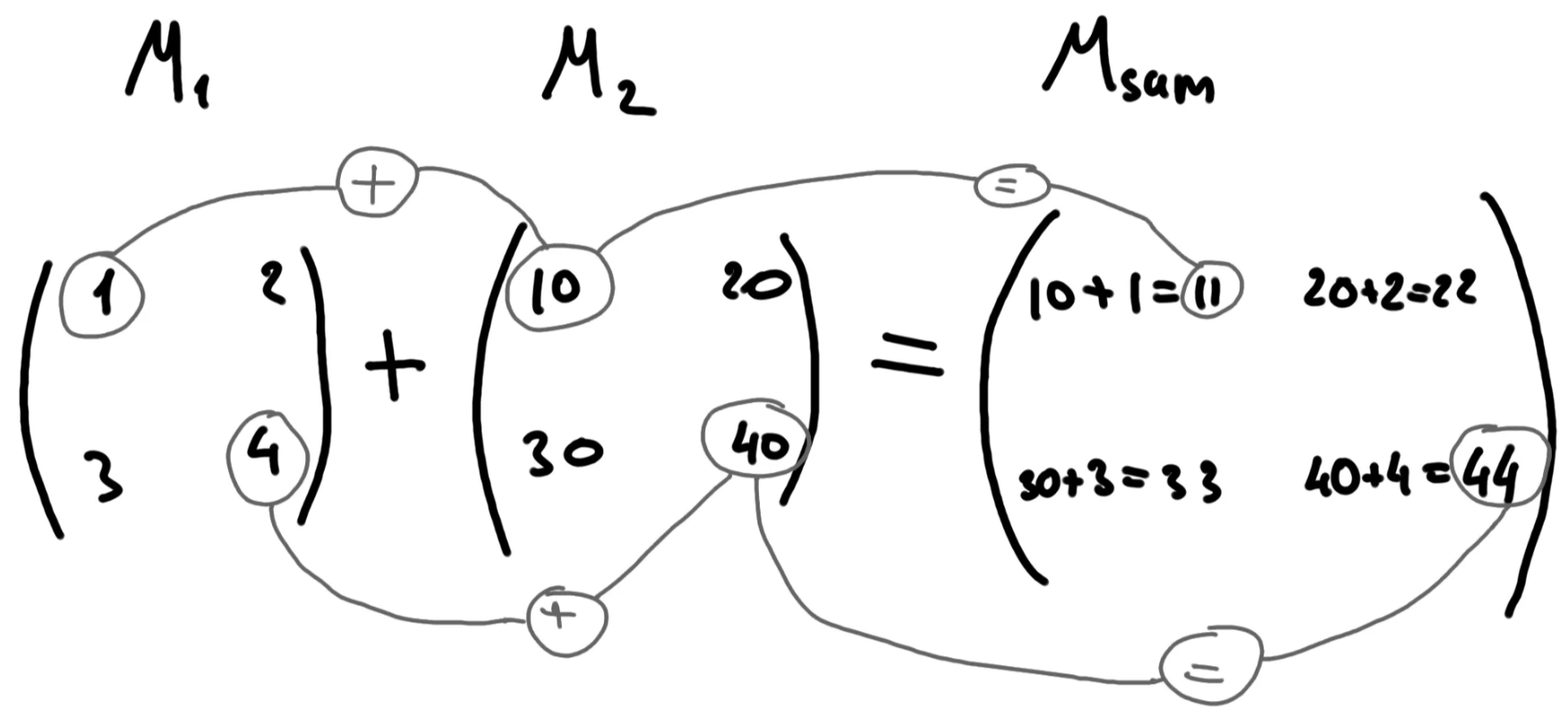
The same approach to add matrices in Python — just use the +
operator:
import numpy as np
a = np.array([[1, 2 ], [3, 4 ]])
b = np.array([[10,20], [30,40]])
sum = a + b
print(sum)
[[11 22]
[33 44]]
sum = a + b
— ifa
andb
are matrices,sum
is also a matrix
Matrices multiplication
This is somewhat tricky. To multiply 2 matrices we have to calculate the sum of row/column value products for each element of the resulting matrix:
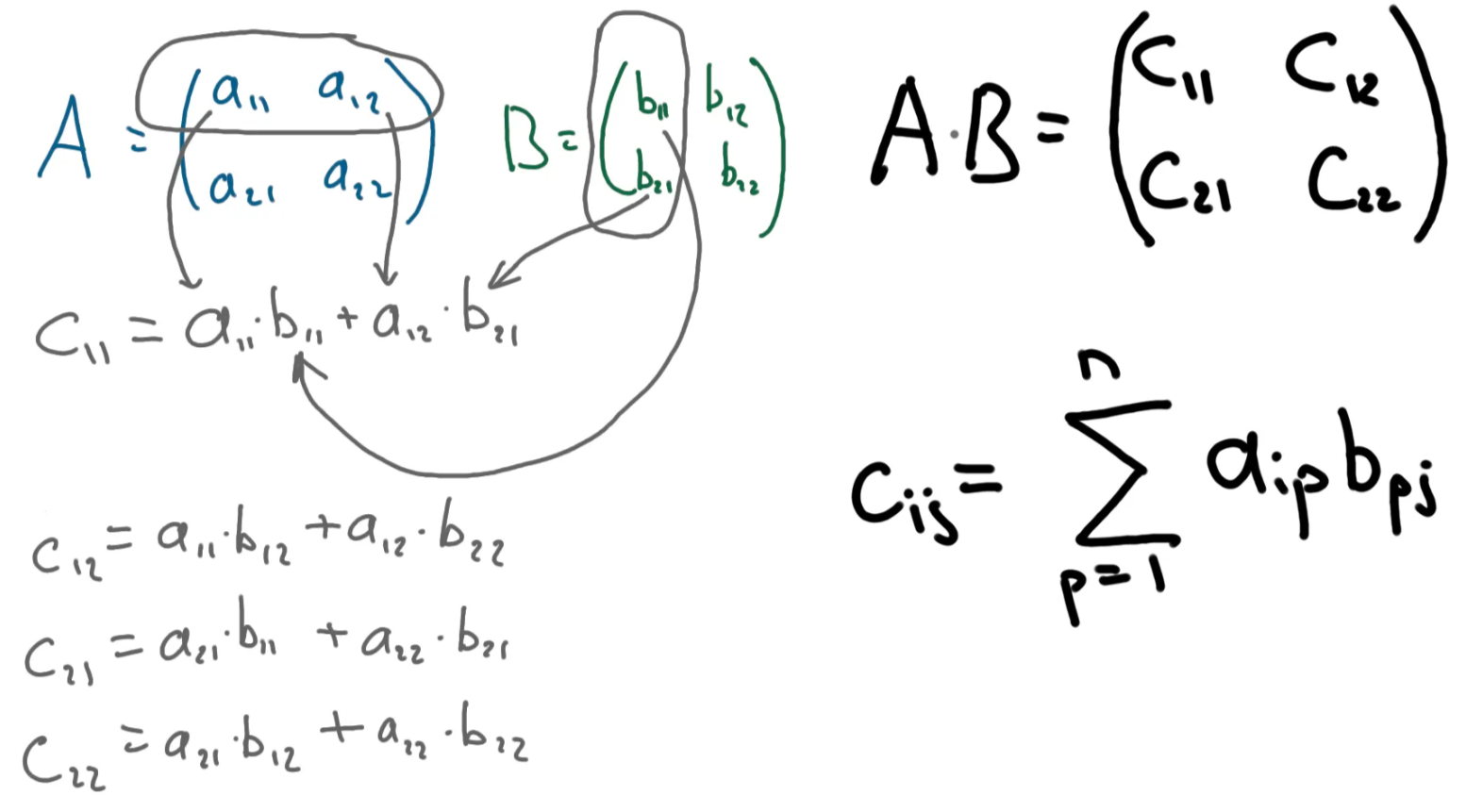
So each element of the new matrix is a sum of the products of corresponding elements of rows from the left matrix and columns from the right matrix. Note, that:
- You can only multiply matrices where the number of left matrix columns is the same as the number of right matrix rows.
- Resulting matrices can be of a different form than source matrices (if they have a different number of rows and columns).
There’s a special @ operator in Python to multiply matrices:
import numpy as np
a = np.array([[1, 2 ], [3, 4 ]])
b = np.array([[10,20], [30,40]])
ab = a @ b
print(ab)
[[ 70 100]
[150 220]]
a @ b
— multiplya
andb
Numpy matrices
Transpose matrix
Transposing matrix is a popular operation as well. To transpose a matrix we just have to change its columns to rows (and rows to columns). In other words — “mirror” it:
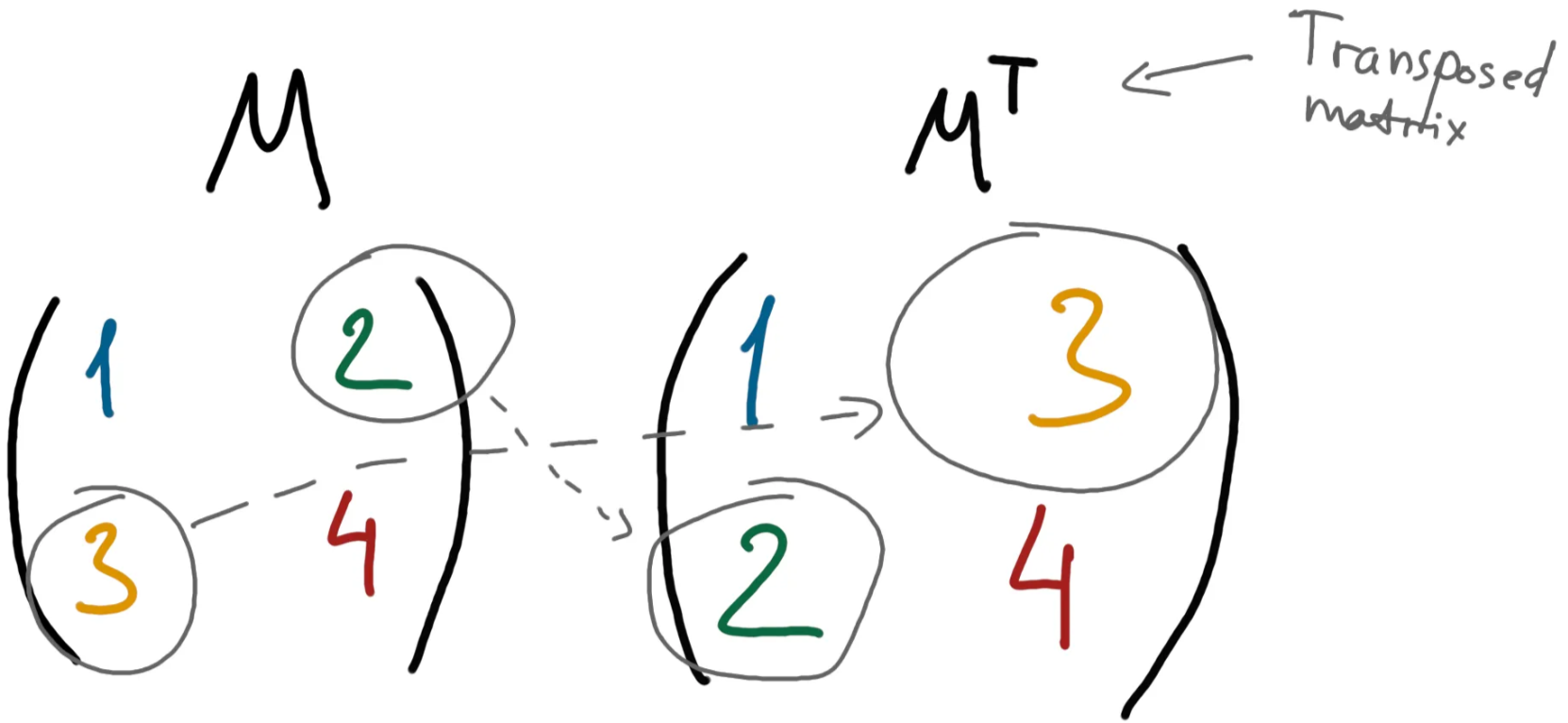
Each Numpy matrix in Python has .T
property which returns transposed matrix:
import numpy as np
a = np.array([[1, 2 ], [3, 4 ]])
print(a.T)
[[1 3]
[2 4]]
Matrix determinant
A matrix determinant is a tool to research a system of equations based on our matrix. It’s calculated in multiple iterations:
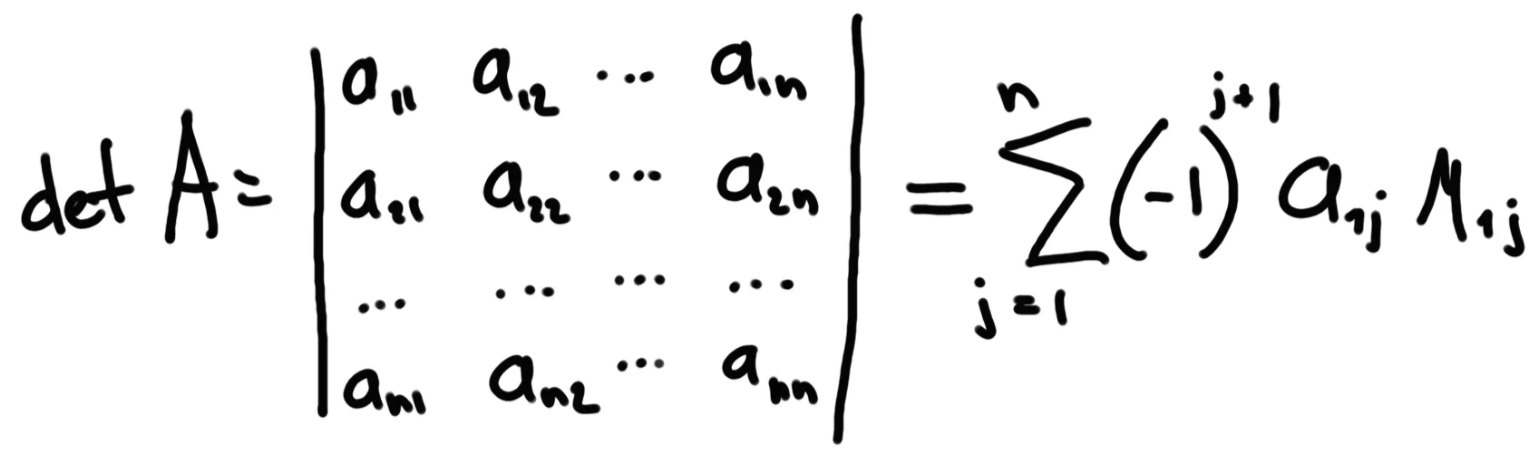
So we have to iterate down to 2x2
matrices from our bigger matrix. For 2x2
matrix determinant is simply calculated as:
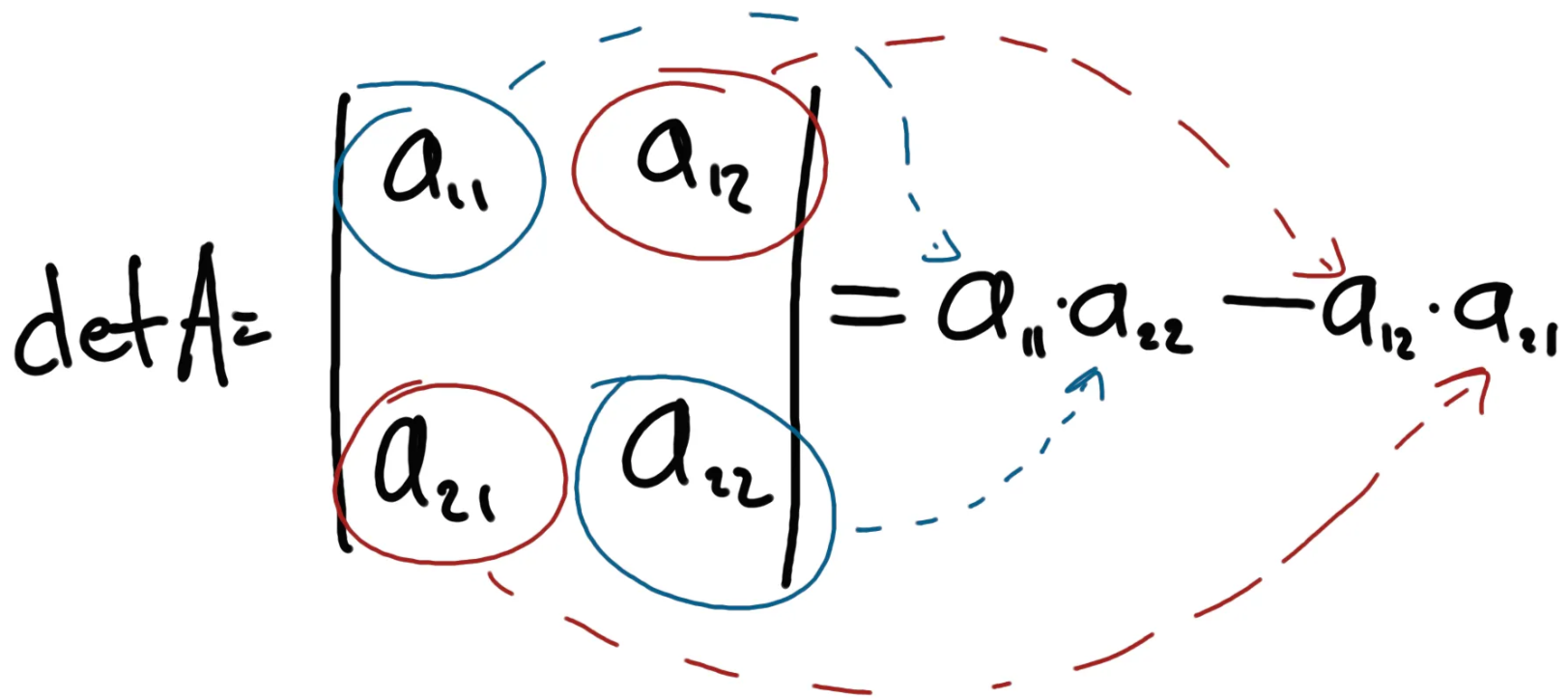
In Python, we can use lingalg.det()
method to calculate matrix determinant:
import numpy as np
a = np.array([[1, 2 ], [3, 4 ]])
det = np.linalg.det(a)
print(round(det))
-2
Matrix rank
Matrix rank is the maximum number of linearly independent columns (or rows) of a matrix and can be calculated in Python:
import numpy as np
a = np.array([[1, 2 ], [3, 4 ]])
rank = np.linalg.matrix_rank(a)
print(rank)
2
Published
2 years ago in #machinelearning
about #math, #matrix and #vector by Denys Golotiuk
Edit this article on Github